Intelligent model optimization
Intelligent model optimization provides automatic refinement of the models you train in an experiment. With intelligent model optimization, the process of iterating feature selection and applying advanced transformations are handled for you. With a well-prepared training dataset that includes all relevant features, you can expect intelligent model optimization to train ready-to-deploy models within a single version.
What is intelligent model optimization?
Intelligent model optimization automates many aspects of the model refinement process. With intelligent model optimization, you can quickly train high-quality models without manually refining feature selection or adjusting your input data.
Using intelligent model optimization
Intelligent model optimization is turned on by default in new ML experiments. You can turn it on or off for each version of the experiment that you run.
After you run an experiment version with intelligent optimization turned on, the results of the optimization can be viewed in the Model training summary. This summary is shown in the Models tab under Model insights. Hover your cursor over underlined terms to view a tooltip with a detailed description.
The Model training summary is different for each model trained in an experiment version.
Model training summary chart for a model, shown in the Models tab
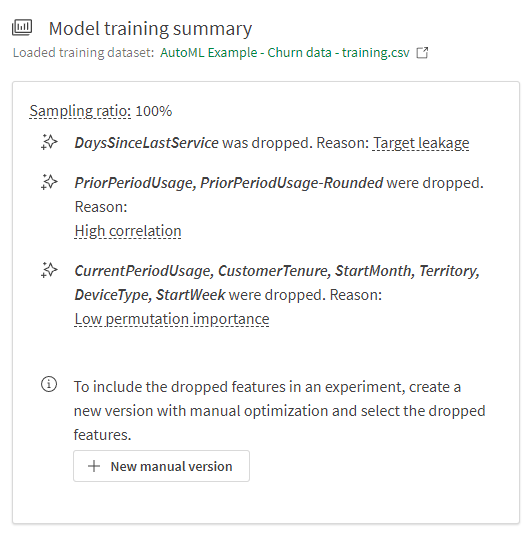
How intelligent model optimization works
With intelligent model optimization:
-
More models are trained than with manual optimization. Feature selection is handled at the model level. This means that unlike manual optimization, each model in a version can have different feature selection.
-
In addition to the automatic preprocessing applied to all models by default, training data is processed with several advanced transformations. These transformations help to ensure that your data is in an optimal format for machine learning algorithms.
-
For quality assurance, a baseline model – a model trained on the entire feature set you configured for the version – is still trained. This helps to check whether the intelligent optimization is, in fact, improving model scores.
-
For larger training datasets, models are trained on a variety of sampling ratios. This helps to speed up the training process. For more information, see Sampling of training data.
Sampling of training data
When you are training models with a large amount of data, AutoML uses sampling to train models on a variety of subsets (sampling ratios) of the original dataset. Sampling is used to speed up the training process. At the start of the training, models are trained on a small sampling ratio. As training continues, models are gradually trained on larger portions of the data. Eventually, models are trained on the entire dataset (a sampling ratio of 100%).
During analysis of model training data, models trained with less than 100% of the training dataset are hidden from some views.
Processing applied during intelligent model optimization
The Model training summary shows how the training data was processed by intelligent model optimization. The following sections contain more detail about each of the items you see in the log.
Feature selection
Intelligent model optimization helps to refine your models by dropping features that can reduce predictive performance. During intelligent model optimization, a feature might be dropped for any of the following reasons:
-
Target leakage: The feature is suspected of being affected by target leakage. Features affected by target leakage include information about the target column that you are trying to predict. For example, the feature is derived directly from the target, or includes information that would not be known at time of prediction. Features causing target leakage can give you a false sense of assurance about model performance. In real-world predictions, they cause the model to perform very poorly.
-
Low permutation importance: The feature does not have much, if any, influence on the model predictions. Removing these features improves model performance by reducing statistical noise.
-
Highly correlated: The feature is highly correlated with one or more other features in the experiment. Features that are too highly correlated are not suitable for use in training models.
In the Data tab within the experiment, you can view insights about dropped features for each model. The Insights also refer to features that were dropped outside of the intelligent model optimization process. For more information about each insight, see Interpreting dataset insights.
Feature transformations
Intelligent model optimization applies a number of technical transformations at the feature level. These transformations process your training data so that it can be used more effectively to create a reliable machine learning model. Feature transformations are automatically applied as needed. In the Model training summary, you are notified when feature transformations are applied, and which features are affected.
Power transform
Feature data often naturally contains distributions with some degree of asymmetry and deviation from a normal distribution. Before training a model, it can be helpful to apply some processing to the data to normalize value distributions if they appear to be overly skewed. This processing helps in reducing bias and identifying outliers.
With intelligent model optimization, numeric features surpassing a specific skew threshold are transformed to have a more normal (or normal-like) distribution using power transforms. Specifically, the Yeo-Johnson Power Transformation is used.
Binning of numeric features
Certain numeric features can contain patterns and distributions that are not easily handled by machine learning algorithms. With intelligent model optimization, this is addressed in part by organizing the data of specific numeric features into different bins depending on their value ranges. Binning is performed so that the features can be transformed into categorical features.
After binning is completed, the new categorical features are one-hot encoded and used in training. For more information about one-hot encoding, see Categorical encoding.
Anomaly detection and handling
Anomalies are data values that appear outside of the range in which you would reasonably expect them to fall. It is not uncommon for there to be some outliers in your training data. Some anomalies might even be desired as a way to reflect real-world possibilities. In other cases, anomalies can interfere with the ability to train a reliable model.
With intelligent model optimization, AutoML identifies potential anomalies. The rows in which the outlier values appear are then handled with an algorithm-powered weighting system. If a value is strongly suspected of being an anomaly, the weighting system reduces the influence of the corresponding row in the training data has on the model.
After your model has been trained, you are notified of the percentage of rows from the original training dataset that were handled as anomalous data.
For more information, see Anomaly detection and handling.
Turning off intelligent optimization
With intelligent optimization turned off, you are optimizing the training manually. Manual optimization can be helpful if you need more control over the training process. In particular, you might want to run a version with intelligent model optimization, then turn the setting off if you need to make a small set of manual adjustments.
Do the following:
-
In an experiment, click
View configuration.
The experiment configuration panel opens.
-
If you have already run at least one version of the experiment, click New version.
-
In the panel, expand Model optimization.
-
Switch from Intelligent to Manual.
Considerations
When working with intelligent model optimization, consider the following:
-
Using intelligent model optimization does not guarantee that your training will produce high-quality models. The dataset preparation and experiment configuration stages are also essential to producing reliable models. If you do not have a well-prepared dataset, or if your configuration is missing key features, your models are not guaranteed to perform well in production use cases. For more information about these stages, see:
-
When intelligent model optimization is turned on for a version, each model from this version will have a separate set of included features. On the other hand, all models from a version trained with manual optimization will have the same set of included features.
-
Intelligent model optimization only uses the features and algorithms you've included in the configuration for the version.
Hyperparameter optimization
Hyperparameter optimization is not available when intelligent model optimization is turned on. To activate hyperparameter optimization, you need to set the model optimization to Manual.
For more information, see Hyperparameter optimization.
Example
For an example demonstrating the benefits of intelligent model optimization, see Example – Training models with automated machine learning.