Navigating the ML deployment interface
When you open your ML deployment, you can perform management and monitoring activites, and use it to create predictions on datasets.
Open an ML deployment from the catalog. There are navigation options for the following:
-
Model approval
-
Deployment information
-
Dataset predictions (batch predictions)
-
Real-time predictions
-
Data drift and operations monitoring
Model approval status
Before the ML deployment can generate predictions, its source model needs to be activated. This process is known as model approval, and helps to control the number of actively used deployed models in the subscription.
If you have the correct permissions, you can activate and deactivate the source model as needed. Otherwise, contact a tenant administrator or other user with sufficient permissions.
See:
Model approval status

Deployment overview
The Deployment overview shows the features used in the model training and details for the deployment.
Overview of the ML deployment
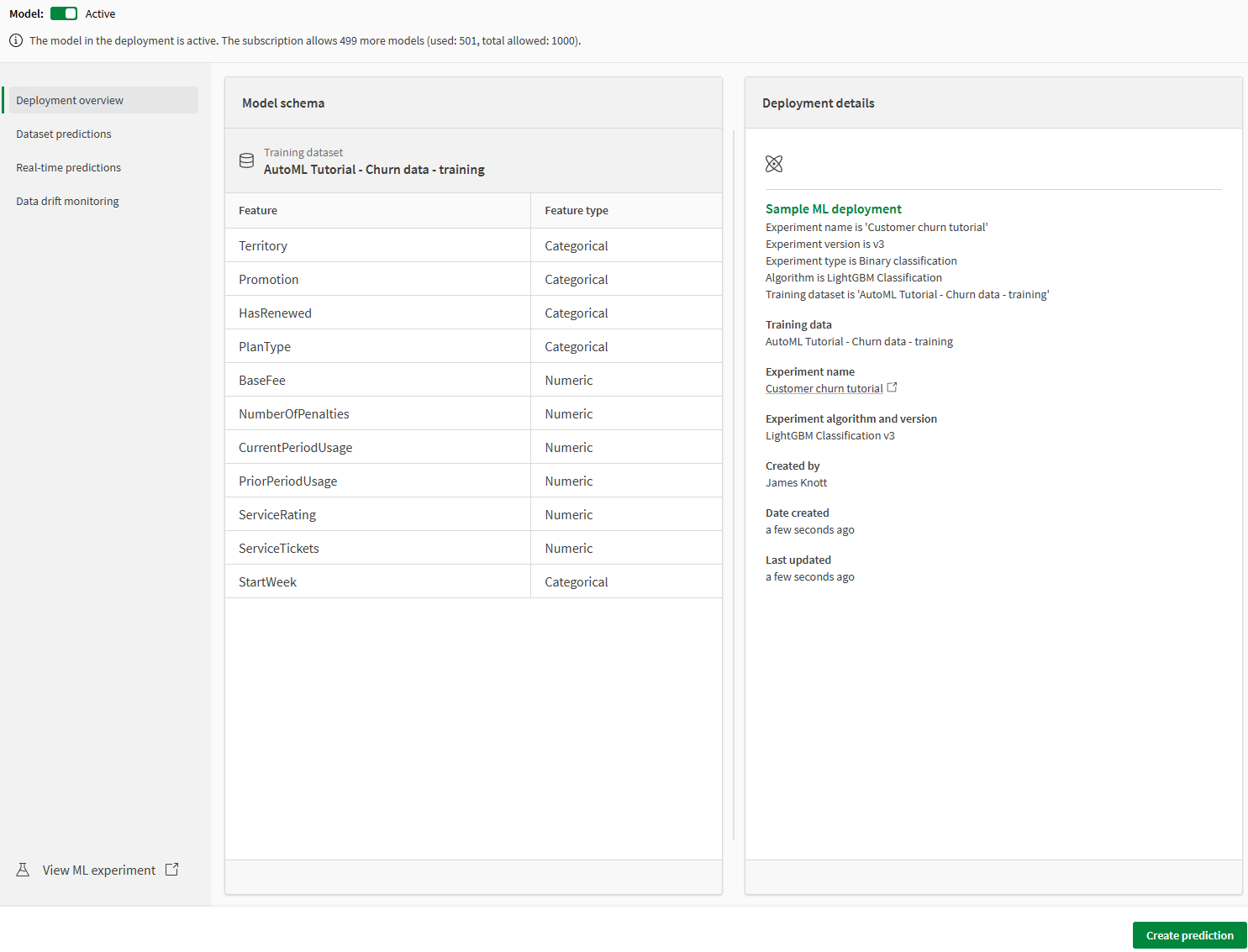
Dataset predictions
In Dataset predictions , you can manage and run batch predictions using the ML deployment. Click Create prediction to create a prediction configuration, from which you run batch predictions. You can have several prediction configurations for an ML deployment.
You can use the Actions menu in the table to:
-
Run predictions from existing configurations
-
Edit and delete configurations
-
Create, edit, and delete prediction schedules for an existing configuration
Dataset predictions with an overview and Actions menu expanded
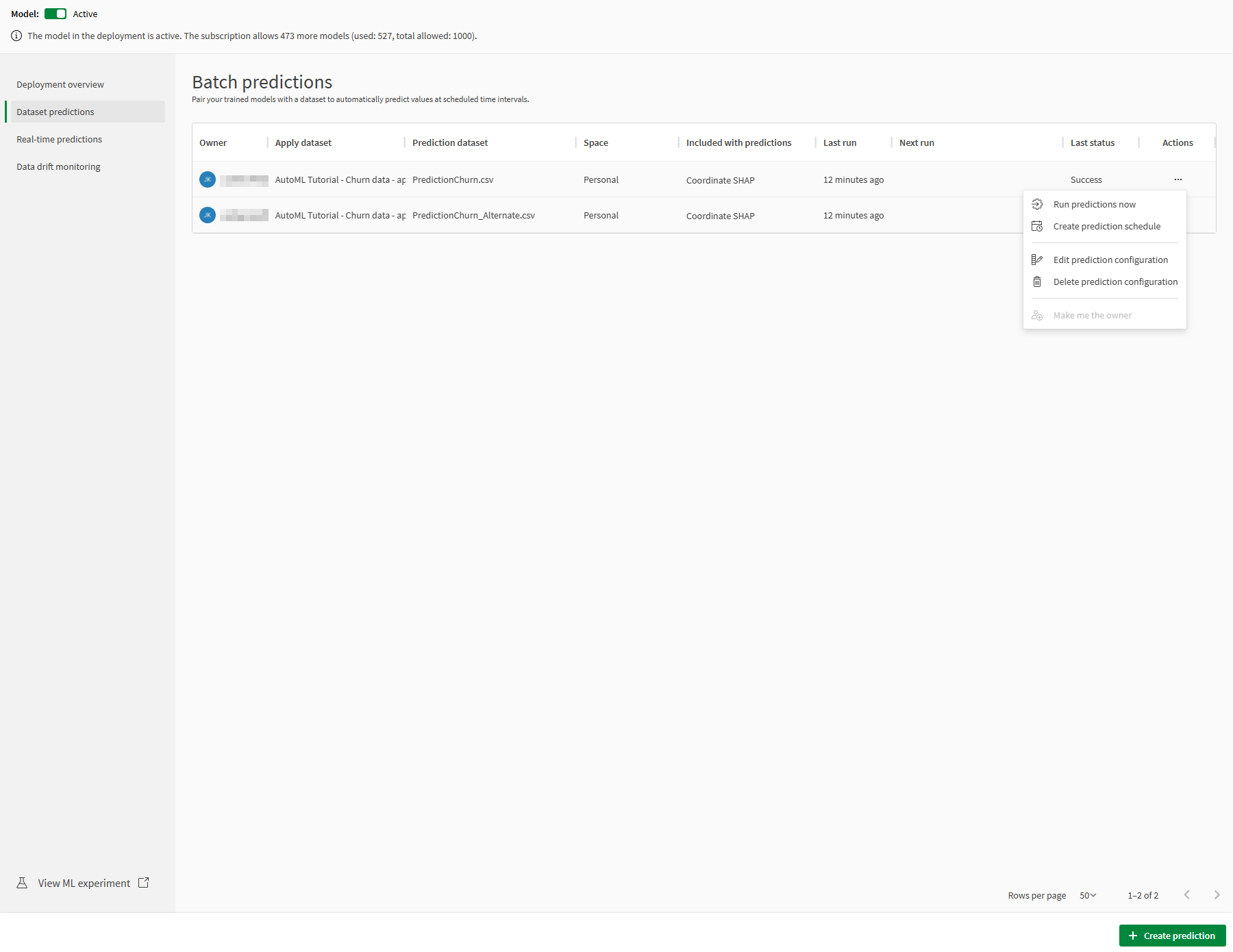
If you select Edit prediction configuration, the Prediction configuration pane is opened.
Dataset predictions with side pane for prediction configuration
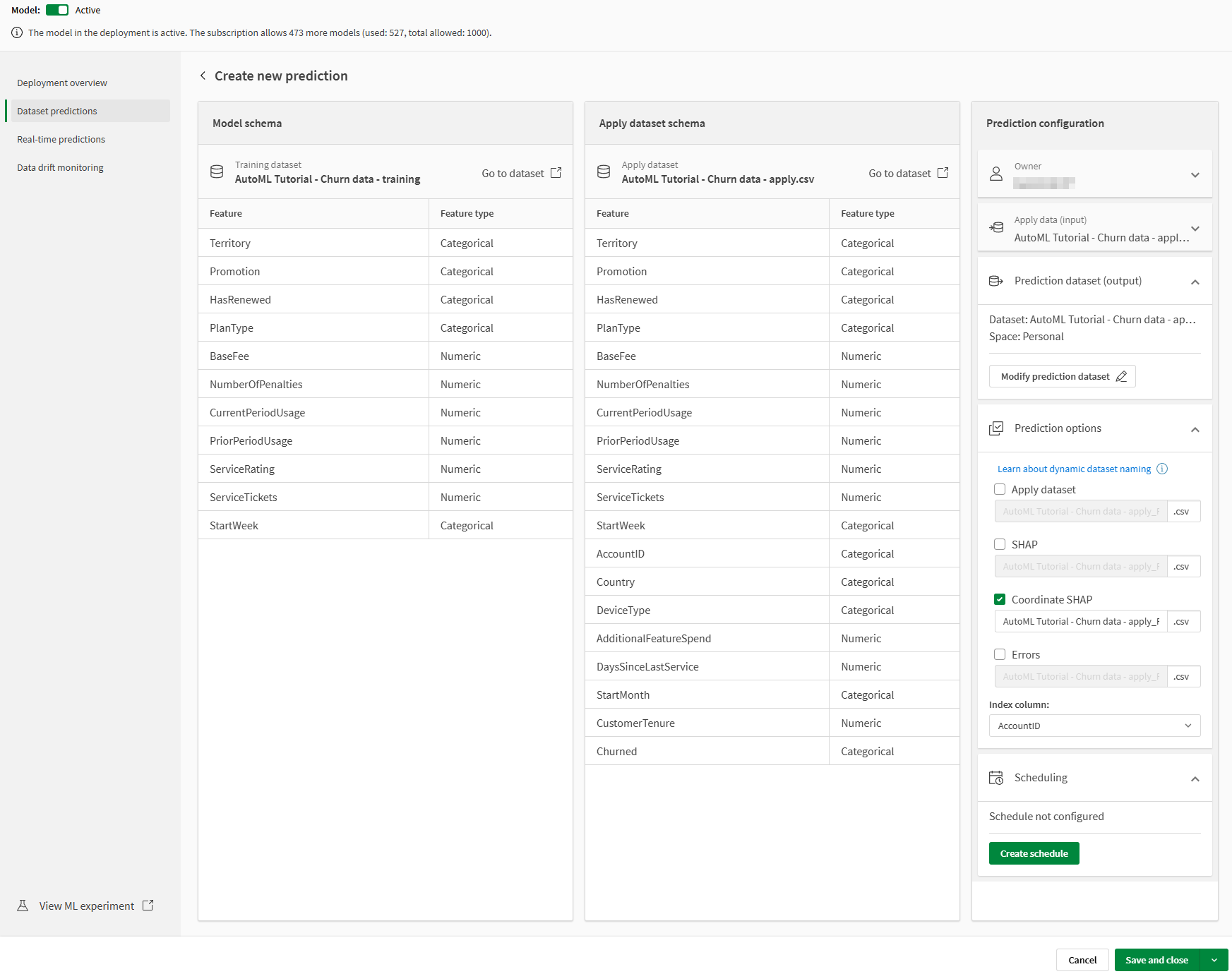
Real-time predictions
The Real-time predictions pane gives you access to the real-time prediction endpoint in the Machine Learning API. If the model in the ML deployment is activated for making predictions, this pane is visible.
For information about creating real-time predictions, see Creating real-time predictions.
The real-time predictions API is deprecated and replaced by the real-time prediction endpoint in the Machine Learning API. The functionality itself is not being deprecated. For future real-time predictions, use the real-time prediction endpoint in the Machine Learning API.
Model monitoring
You can monitor data drift and operations for the ML deployment. To perform model monitoring, open the Data drift monitoring pane.
With data drift monitoring, you can assess changes in the distribution of features in the source model. When significant drift is observed, it is recommended that you retrain or re-configure your model to account for the latest data, which may indicate new patterns in data trends.
For more information, see Monitoring data drift in deployed models.
With operations monitoring, you can view details about how the ML deployment is being used, such as how many prediction events succeed or fail, and how prediction events are typically triggered.
For more information, see Monitoring deployed model operations.
Data drift monitoring pane in AutoML
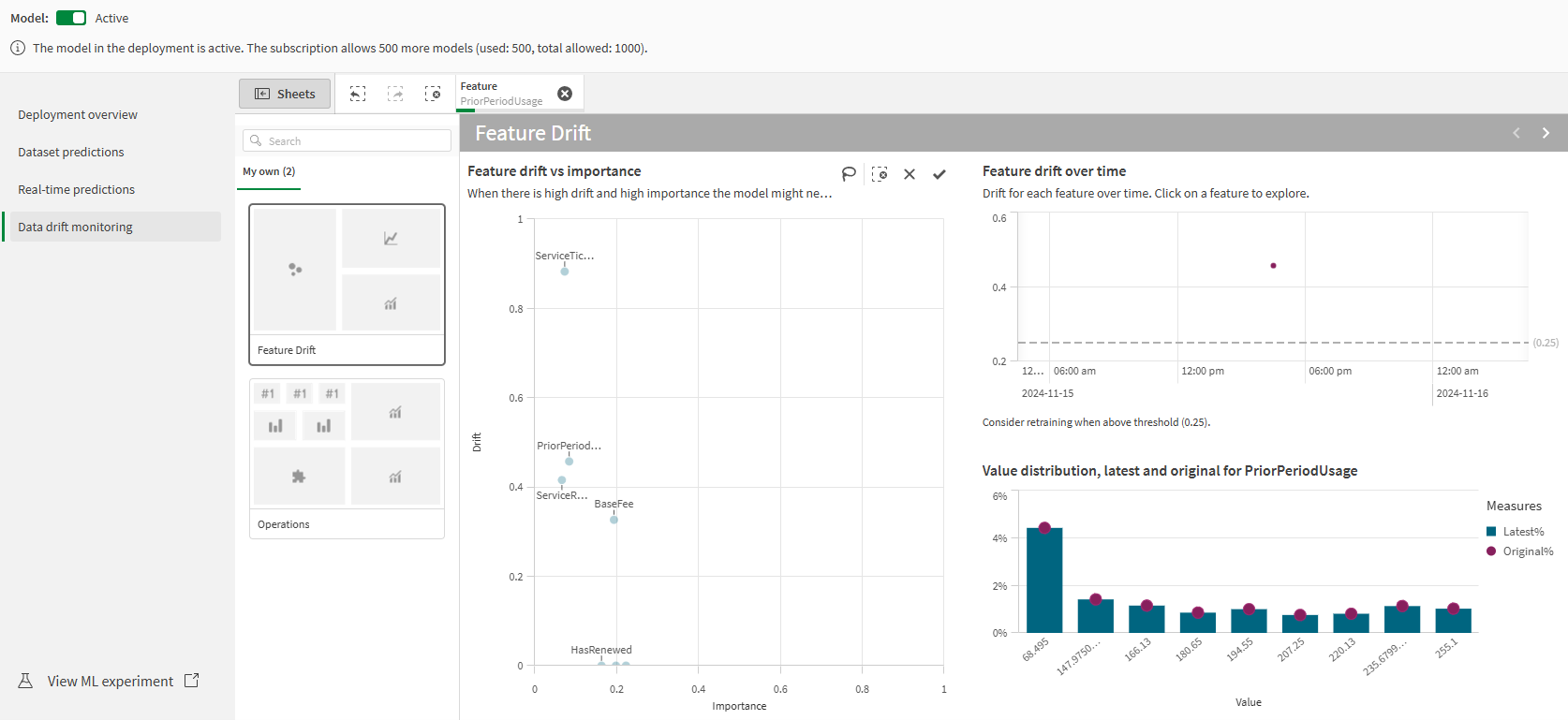
View ML experiment
Click View ML experiment in the bottom left corner of the page to open the ML experiment from which the ML deployment was created.
Overview of the ML deployment
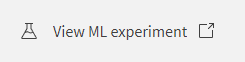